Customising AI Chatbots for Multilingual Mothers' Mental Health
This project was my HCI master’s dissertation at UCL. It explores how AI chatbots can be customised to support multilingual mothers by addressing challenges in accessing mental health support in their native languages and dialects. This study underscores the importance of training large language models (LLMs) with diverse linguistic data to make mental health support more equitable and accessible for all.
Introduction
Mental health support is crucial for mothers, but multilingual mothers often encounter significant barriers when trying to access appropriate care. Language differences, cultural misunderstandings, and stigma can hinder effective communication and support. My research examines how AI chatbots can be customised to overcome these challenges, ensuring that mental health interventions are inclusive and effective for users from diverse linguistic backgrounds. Many mental health resources are primarily available in English, which puts non-English speakers at a disadvantage. Therefore, my research focuses on designing linguistically inclusive AI chatbots that allow for seamless language switching and adaptation to different dialects. I evaluated the effectiveness of these chatbots through user testing.
.png)
Research Question
How can conversational AI systems be designed to support the language needs of multilingual mothers in self-managing their mental health?
.png)
Methodology
To investigate this, I conducted a co-design study involving multilingual mothers. The research followed a participatory approach, engaging participants in four structured co-design sessions to ensure their needs and preferences were central to the chatbot design process.
.png)
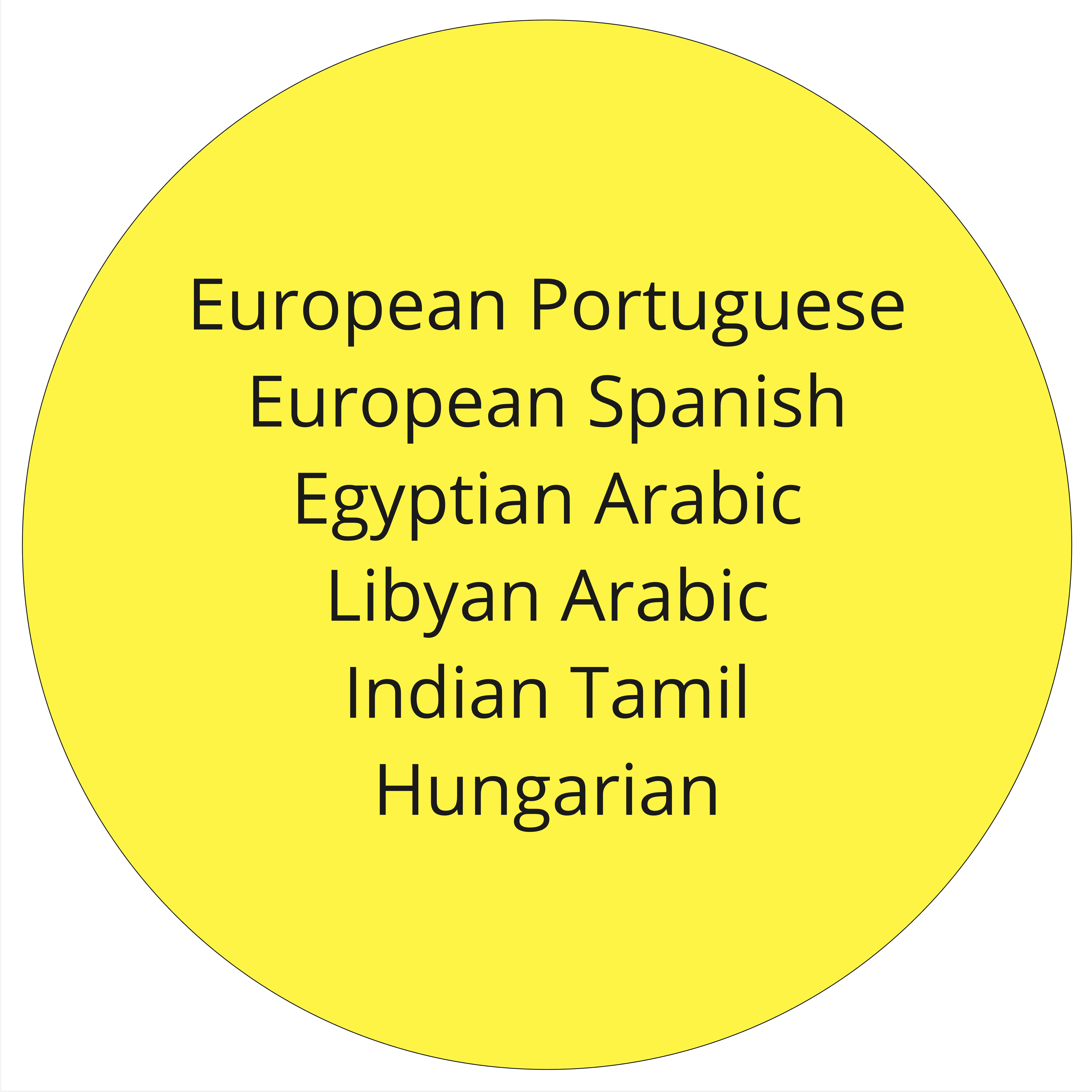
Study Design
- Participants: Six multilingual mothers from different linguistic backgrounds.
- Data Collection: Qualitative methods including interviews, storyboarding, and co-design workshops.
- Analysis: Reflexive thematic analysis to identify key linguistic and cultural needs.
- Tools Used: Miro for ideation, Canva for co-design materials, and OpenAI's GPT-4o for chatbot development.
.png)
Co-Design Sessions
Session 1: Understanding Mental Health Needs and Language Preferences

The focus of this session was to identify the mental health challenges faced by multilingual mothers and to explore their language preferences for emotional expression. The research involved understanding the participants' mental health needs and linguistic preferences through the use of Miro and six AI-generated visual cards. These visual aids served as prompts for discussions about mental health, coping strategies, and language use.
Participants choose which topic they want to discuss first, creating a participant-driven experience. Open-ended questions encouraged reflection on how language impacts emotional expression and help-seeking behaviours. This initial session established trust, offered valuable insights into the role of language in mental health, and set the stage for future sessions.
Tools Used: Miro for virtual collaboration, Canva for creating visual activity cards.
.png)
Session 2: Storyboarding Daily Routines and chatbot Interaction Points

Participants mapped their daily routines using Miro and a storyboarding toolkit, focusing on their emotional experiences and patterns of language switching. They created storyboards that represented their daily schedules, highlighting stress points and moments of language switching. These visual representations reflected their routines.
Participants annotated their emotions in their storyboards and identified contextual factors influencing their language choices. For instance, they noted how they used their first language for emotional comfort at home while using English in professional settings. They also pinpointed potential integration points for a chatbot, expressing their preferences for text or voice formats.
The session aimed to identify key moments in participants' daily lives when AI chatbots could provide mental health support. The storyboard creation process helped participants understand how chatbots could be used in daily life.
Overall, the session provided valuable insights into the optimal times for chatbot engagement. Participants found the creative and collaborative process enjoyable, allowing them to reflect on their routines from a new perspective.
Tools Used: Miro for interactive storyboarding and guided discussion prompts.
.png)
Session 3: Co-Designing AI Chatbot Prompts

Participants customised their chatbot by selecting emotional regulation techniques and tailoring the language and conversational styles. First, they explored techniques such as gratitude journaling and breathing exercises using AI-generated visual cards, choosing those most relevant to their well-being.
Next, they personalised the chatbot's persona by defining their preferred languages, dialects, and culturally appropriate expressions. They completed a table designed to define the chatbot persona, identity and to outline the conversation flow for greetings, emotional support, and expressions of empathy. Participants contributed common phrases, greetings, and empathetic expressions in their native languages to be integrated into the chatbot's responses.
The session generated excitement about the next gathering, at which participants would have the opportunity to test their customised chatbots.
Tools: Miro, AI-generated regulation cards
.png)
Between Sessions 3 & 4

Between the third and fourth co-design sessions, I developed personalised chatbot prototypes based on participants' preferences. By using data from previous sessions—such as emotional regulation techniques, language preferences, and cultural expressions—I created a customised prompt for each participant. I created the backend & chatbot functionality using OpenAI GPT-4o API and integrated the customised prompts I made for each participant into the code, all stored in Git Hub. A user-friendly interface was created with Streamlit, allowing participants to interact with their chatbot during the final session. The chatbots are all set for the last testing session.
.png)
Session 4: Testing and Evaluating the Customised AI Chatbot
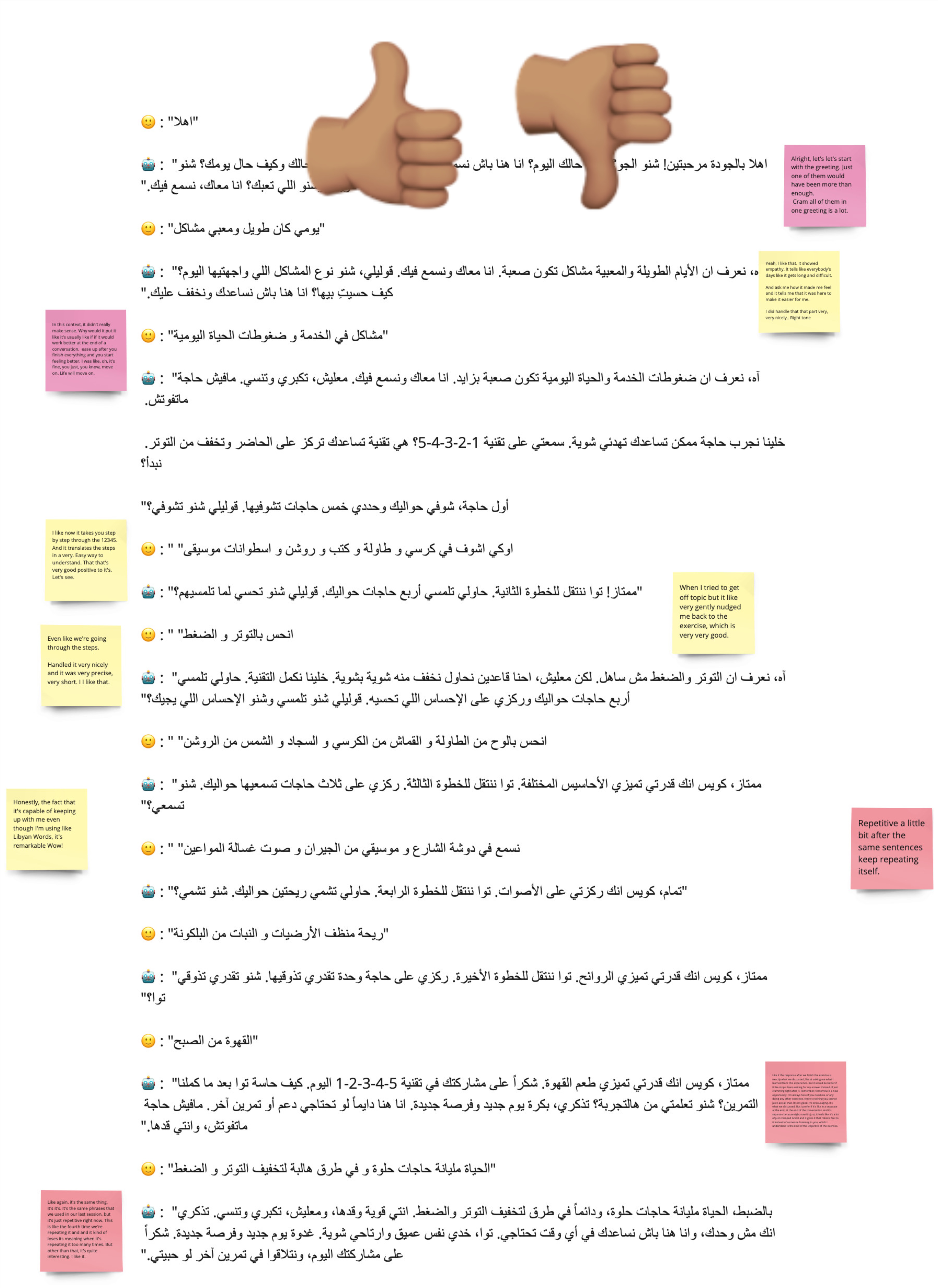
The final session evaluated how effectively the chatbot addressed participants' language preferences and dialect needs. Participants interacted with the AI chatbot, providing real-time feedback on tone, linguistic accuracy, and cultural relevance, as well as suggesting improvements.
During the interaction, participants used the think-aloud method to give immediate feedback on their experiences. Afterward, they reviewed their conversation logs for deeper reflection and analysis, identifying strengths and areas for improvement in fluency, accuracy, flow, and cultural fit. The goal was to assess the chatbot's effectiveness in delivering mental health support that aligns with linguistic and cultural needs. This session aimed to gather feedback on the chatbot's ability to support various languages, dialects, and cultural nuances.
Tools Used: OpenAI’s GPT-4o API, Streamlit and Miro.
.png)
Data Analysis
.jpg)

Data Analysis:
A bottom-up reflexive thematic analysis revealing insights directly from participants' experiences:
1- Individual Analysis: Reviewed each participant's data to understand personal language and mental health experiences.
2- Horizontal Analysis: Compared data across participants to identify shared themes and patterns.
3- Cross-Cutting Themes: Analysed Cross-Cutting Themes, the recurring patterns to uncover broader trends in multilingual experiences.
4- Narrative Development: Synthesising the findings into actionable recommendations for inclusive AI chatbot design.
.png)
Key Findings
Linguistic Challenges Faced by Multilingual Mothers
Native Language Preference: Participants overwhelmingly preferred mental health support in their native language, as it allowed for more authentic emotional expression.
.png)
Cognitive Load and Restrictions: Expressing emotions in a non-native language imposed an additional mental burden, making it difficult for participants to articulate their thoughts and feelings effectively. Participants reported feeling restricted in communicating their emotions when using a second language, sometimes leading to detachment or frustration.
P6 Hungarian, "I will never express myself as well in English as in Hungarian because that is my language"
.png)
Code-Switching: During emotionally intense moments, participants instinctively reverted to their native language, which felt more natural and comfortable.
P5 European Spanish, "If I’m driving and something happens, the first thing that comes to my mind is Spanish. It’s automatic when I need to shout or express strong emotions"
.png)
Lexical Gaps: Many participants struggled to express mental health concepts in their native languages due to the absence of equivalent terms, often resorting to English words instead. For example, "mindfulness" was frequently referenced in English as there was no widely recognised translation in their languages.
P4 Egyptian Arabic, "A word like mindfulness is tricky to translate into Arabic. It’s not commonly used in spoken Egyptian dialect. People in my community are more familiar with it in English through social media or self-help books"
.png)
Emotional Resonance: Even when fluent in English, participants found that strong emotions were best expressed in their native tongue, reinforcing the importance of linguistic inclusion in mental health tools.
These findings underscore the need for AI-powered mental health tools that accommodate linguistic diversity, support code-switching, and incorporate culturally relevant expressions to foster authenticity, engagement, and emotional comfort.
.png)
Benefits of Using Custom AI Chatbots in one's native Languages
Effective Emotional Regulation Support : Custom AI chatbots provided participants emotional guidance tailored to their linguistic and cultural backgrounds. The chatbots facilitated emotion regulation techniques in participants’ native languages, making support more accessible and relatable. One participant noted that the chatbot’s phrasing resembled everyday speech and professional counselling techniques, enhancing its authenticity by mimicking active listening strategies.
P4 Egyptian Arabic, "I am amazed. It was excellent! The use of Egyptian Arabic was really impressive.The way of phrasing things is very close to what I use in my counselling sessions"
.png)
Empathetic Conversation Management: Participants appreciated the chatbot’s empathetic and engaging responses. They valued the chatbot’s ability to acknowledge challenges and promote gratitude, contributing to a more reflective and positive mindset. Several participants expressed enthusiasm for using the chatbot immediately, emphasising its potential to provide timely mental health support.
P1 Libyan Arabic, "It told me everybody’s days sometimes get long and difficult"
P4 Egyptian Arabic, "It helped me realise there are three or four things I should be grateful for"
.png)
Cultural Sensitivity & Trust: Mental health stigma influences help-seeking behaviours in some cultures. AI chatbots must incorporate culturally adapted responses to enhance user trust and engagement.
.png)
User Interaction Preferences: Participants preferred voice-based interaction over text due to its natural and emotionally resonant experience. AI chatbots should provide personalised and contextual responses instead of generic, scripted text.
These insights demonstrate that custom AI chatbots when designed with linguistic and cultural considerations, can effectively support mental health needs and closely replicate professional counselling approaches.
.png)
Challenges of Using Custom AI Chatbots in Non-English Languages
Repetitive and Unnatural Conversational Flow: Participants noted that repetitive phrasing and awkward pacing disrupted the natural flow of interactions, diminishing the conversational quality with repeated instructions and greetings.
P2 European Portuguese, "There was quite a bit of repetition. Hearing the same prompt over and over made the conversation feel less natural"
.png)
Inconsistencies in Language Style: The chatbot faced challenges in maintaining a consistent language style in some languages. It often mixed formal and casual tones or used unnatural translations, which disrupted conversational flow and reduced relatability, especially in dialect-specific and culturally nuanced contexts.
P3 Indian Tamil, noted instances where the chatbot mixed formal and casual language or included English words written in Tamil letters, which disrupted the natural conversational flow
.png)
Cultural and Contextual Irrelevance: Participants stressed the importance of culturally sensitive context-aware interactions. They suggested using more casual language and slang to create a conversational tone that aligns with everyday communication.
P6 Hungarian, pointed out that the chatbot’s language felt too formal and professional, suggesting more casual expressions and slang to create a conversational tone that resonates better with everyday communication
.png)
These challenges highlight the need to enhance conversational flow, ensure linguistic consistency across different languages and dialects, and incorporate cultural relevance to make them intuitive, relatable, and effective for diverse users.
.png)
The Critical Role of Linguistic Diversity in AI-Powered Mental Health Support
As AI-driven mental health interventions continue to expand, the need for linguistically and culturally inclusive chatbots has never been more urgent. Language is not merely a means of communication—it is deeply tied to emotional regulation, identity, and cultural expression. Multilingual individuals, particularly mothers managing mental health challenges, require support that reflects the complexity of their linguistic realities.
.png)
Why Linguistic Diversity Matters in AI Mental Health Tools
Mental health support is highly personal, and language choice is crucial in how individuals express and process emotions. Research findings highlight that multilingual users frequently switch between languages depending on their emotional state, social context, and cognitive ease. This fluidity is even more pronounced for multilingual mothers as they navigate multiple linguistic and cultural identities while managing the stresses of parenting.
However, current AI chatbots and mental health support tools remain heavily English-centric, often failing to accommodate diverse users' nuanced linguistic and cultural needs. Without proper multilingual adaptation, these tools risk alienating non-English speakers or providing incomplete and ineffective support.
.png)
The Impact of Multilingual AI Chatbots on Mental Health Support
AI chatbots have emerged as an accessible, scalable solution for delivering mental health support, particularly in situations where traditional therapy may be inaccessible due to cost, stigma, or logistical barriers. Multilingual mothers in particular—who often struggle with time constraints, cultural barriers, and the emotional burden of parenting—benefit from AI chatbots that provide on-demand, language-adaptable mental health support.
Participants in research studies likened chatbot interactions to conversations with a close friend, valuing the ability to engage in mental health discussions in their preferred language without the fear of judgment. Furthermore, in cultures where mental health remains a stigmatised topic, chatbots offer a private, non-threatening space for individuals to express their emotions and seek guidance.
Additionally, AI chatbots that communicate in a user's native language are crucial in normalising and familiarising mental health terminology. Many mental health concepts are predominantly discussed in English, which creates a gap in understanding for non-English speakers. By introducing these terms in native languages, chatbots can bridge this knowledge gap, making mental health discussions more accessible and culturally relevant. Over time, this can reduce stigma and increase awareness in communities where mental health is still a sensitive or taboo subject.
.png)
Key Design Considerations for Multilingual AI Chatbots
To create effective, inclusive AI mental health tools, several key design principles must be prioritised:
Multilingual Adaptation: AI chatbots should seamlessly switch between languages based on user preferences and conversational flow, reflecting how multilingual individuals naturally communicate.
Culturally Inclusive Prompts: Chatbot responses must be contextually aware, ensuring that cultural sensitivities, idiomatic expressions, and localised mental health terminology are accurately represented.
Empathy & Active Listening: AI systems should mimic human-like empathy, using conversational cues that provide validation, encouragement, and emotional support.
Voice-First Design: Many users, particularly multilingual individuals, prefer verbal expression over text-based communication. Voice-based AI interactions improve emotional resonance, accessibility, and user engagement.
Bridging the Linguistic Gap in AI-Language ModelsThe effectiveness of AI-powered mental health tools hinges on how well Large Language Models (LLMs) are trained to handle diverse linguistic and cultural needs. Diverse representation in training datasets is critical to preventing bias and ensuring that AI chatbots can accurately understand, interpret, and respond to users in multiple languages. AI mental health interventions risk perpetuating exclusion and inequity in digital health services without careful consideration of linguistic variation, dialects, and cultural nuances.Furthermore, by introducing mental health discourse in native languages, AI chatbots can help destigmatise mental health discussions in communities where such conversations are still avoided. This increased accessibility can drive societal shifts, making mental health care more inclusive and approachable for all.
.png)
Conclusion
Linguistic diversity should not be an afterthought in AI-powered mental health solutions. If AI chatbots are to serve diverse, global populations, they must be designed with language fluidity, cultural awareness, and emotional intelligence at their core. By enabling seamless language switching, embedding cultural nuance, and refining empathy-driven AI interactions, we can create equitable, effective, and human-centred mental health interventions that truly support multilingual users.As AI technology advances, designers, researchers, and engineers are responsible for ensuring that digital mental health tools empower rather than exclude. The future of AI-driven mental health care depends on breaking linguistic barriers and fostering inclusivity, making support accessible for all—regardless of language or cultural background.
.png)